Elasticsearch is one of the most popular analytics platform for large
datasets. It is useful for a range of use-cases ranger from log
aggregation, business intelligence as well as machine learning.
Elasticsearch is popular because of its simple REST based API which
makes it trivial to create indices, add data and make complex queries.
However, before you get up and running building your dataset and running
queries you need to setup a elasticsearch cluster, which can be a
somewhat daunting prospect. Today, we look at how Rancher Catalogs make
it trivial to setup a scalable, highly available Elasticsearch cluster.
Assuming you already have a Rancher Cluster up and running, getting
Elasticsearch running on your cluster is a simple matter of browsing to
Catalog in the top menu and searching for Elasticsearch. There are two
versions of the Elasticsearch catalog. We are assuming that you are
using 2.x, the latest stable release version. To launch the stack from
the cluster, select View Details, and in the subsequent screen choose
a Stack Name, Cluster Name and select launch.
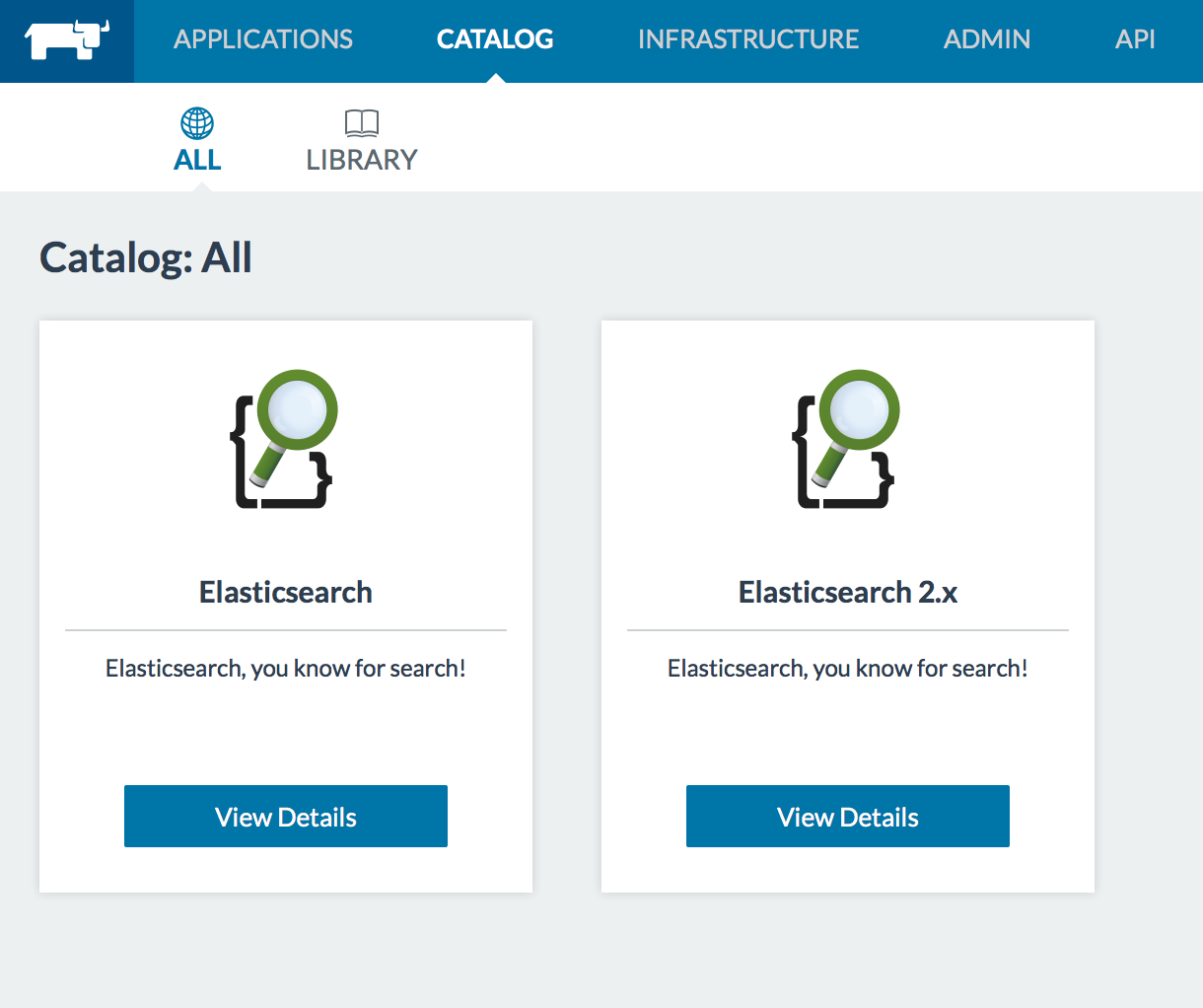
The stack should launch the following services;
kopf, client(s),
datanode(s) and master(s). The kopf container provides a web interface
to manage your elasticsearch cluster. Datanodes store the actual
indices. The master node runs cluster management tasks and the client
nodes originate and coordinate your searches and other operations.
Initially, your Elasticsearch cluster will have one container of each
type (master, client and datanodes have two sidekick containers).
However, you can scale out each of those components based on query load
and the size of the indices. Note that you need different physical hosts
for each datanode container to function correctly. Hence, you may have
to register more Rancher compute nodes.
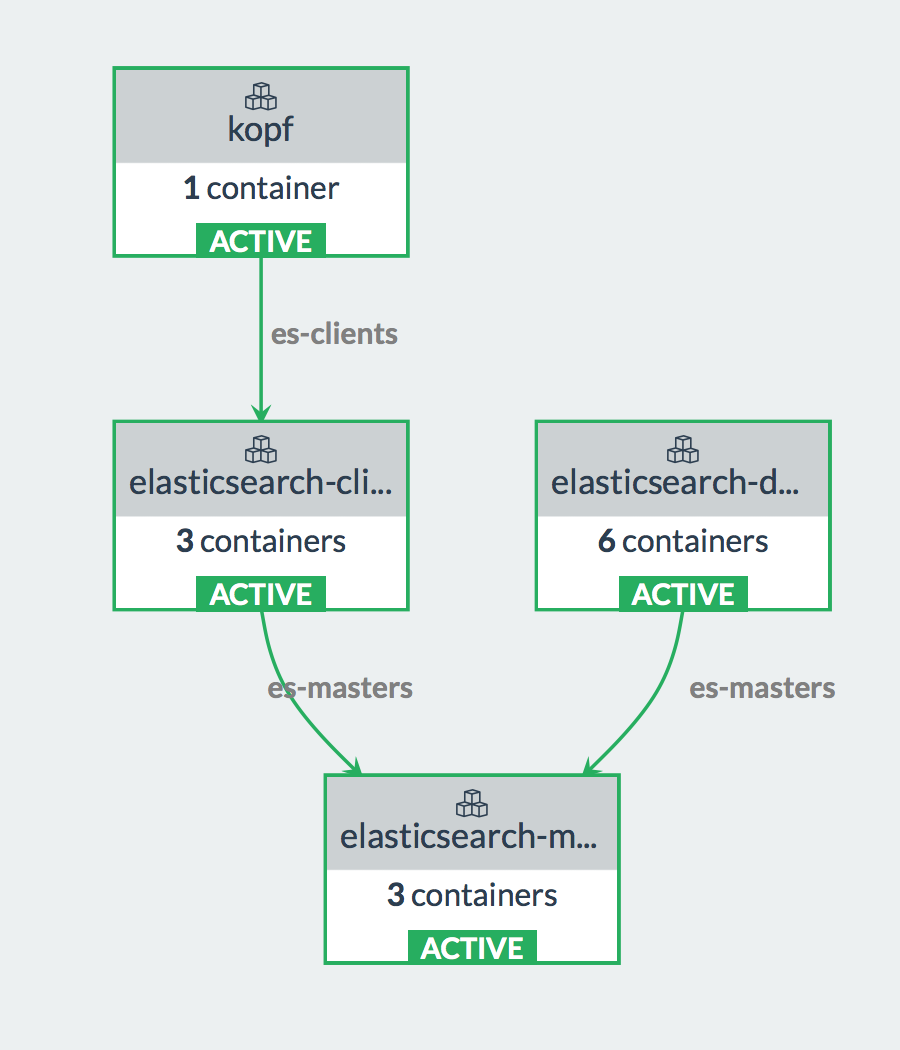
Once all your containers are active, you can bring up the kopf interface
by browsing to the host running the kopf container. If you click on the
nodes tab, you will see the various components I mentioned above
listed. As you can see, I have launched a second data node in order to
provide redundant storage for my indices. As we will see shortly, when
creating indices, we can control the number of data shards and copies of
each shards. This will help provide redundancy as well as speedup query
processing.
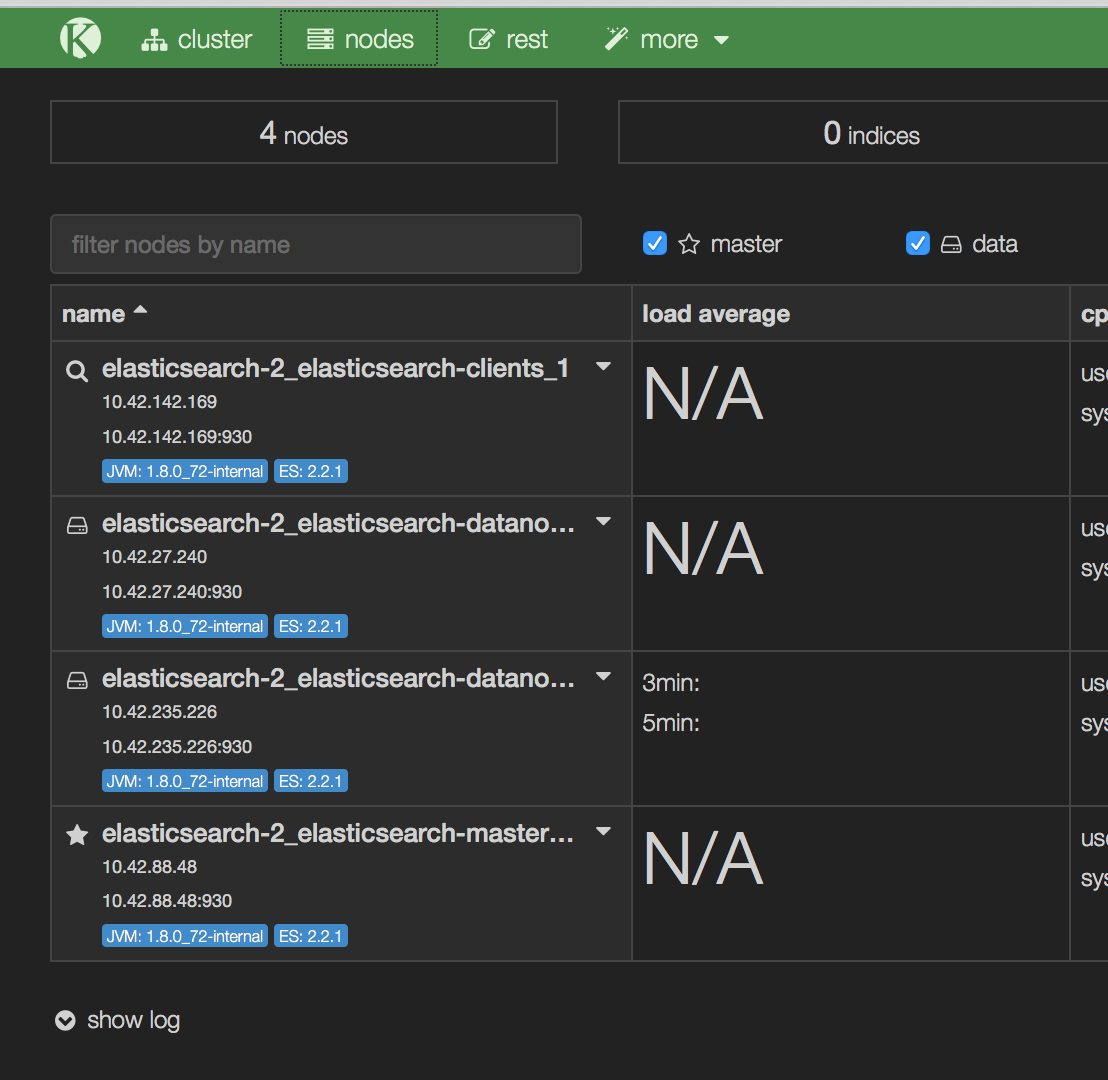
From the menu on the top of kopf, select more and then create index.
In the resulting screen, you will be asked to enter the Index Name,
the Number of Shards and the Number of replicas. The defaults for
these are 5 shards and 1 replica respectively. The number of shards and
replicas to setup for an index is highly dependent on the data set and
query model. The number of shards help spread data onto multiple nodes
and allow parallel processing of queries. Hence, if you only have a
single datanode then you will not see much benefit from multiple shards.
In addition, if you expect the data to grow rapidly you may want to have
more shards so that you can add nodes later and have data move to those.
Another thing to keep in mind is that elastic search recommends a max
heap size of 32 GB and hence, a max shard size should be about that size
so that it can be kept in memory as much as possible.
Replicas, on the other hand, are less related to datasize and more to
redundancy and performance. All queries for your index need to look at
one copy of each shard. If you have multiple copies of the shard, the
data is resilient to one node going down. Furthermore, with multiple
copies, the query load for a given shard is split between multiple
nodes. Having multiple replica only makes sense when you have more than
one data container/node in your cluster, and becomes more important as
you scale larger and larger cluster sizes.
As an example, lets define an index called movies with 2 shards and 2
replicas. Now select the rest tab from the top menu so that we can add
some documents to our index and test some queries. Elasticsearch is
schema free so we can add free form data into our index as long as it is
valid JSON. Update the path field to /movies/movie/1. The format of
the path is /INDEX_NAME/TYPE/ID where movies is the index we just
created, movie is the name we are giving to the type of document we are
about to submit and id is a unique ID for the document within the index.
Note the ID is optional, and if you skip this from the path, a random ID
will be created for your document. Once you have added the path, select
POST as the method, enter your JSON document in the bottom text field
and hit send. This will add the document to the index and send you a
confirmation.
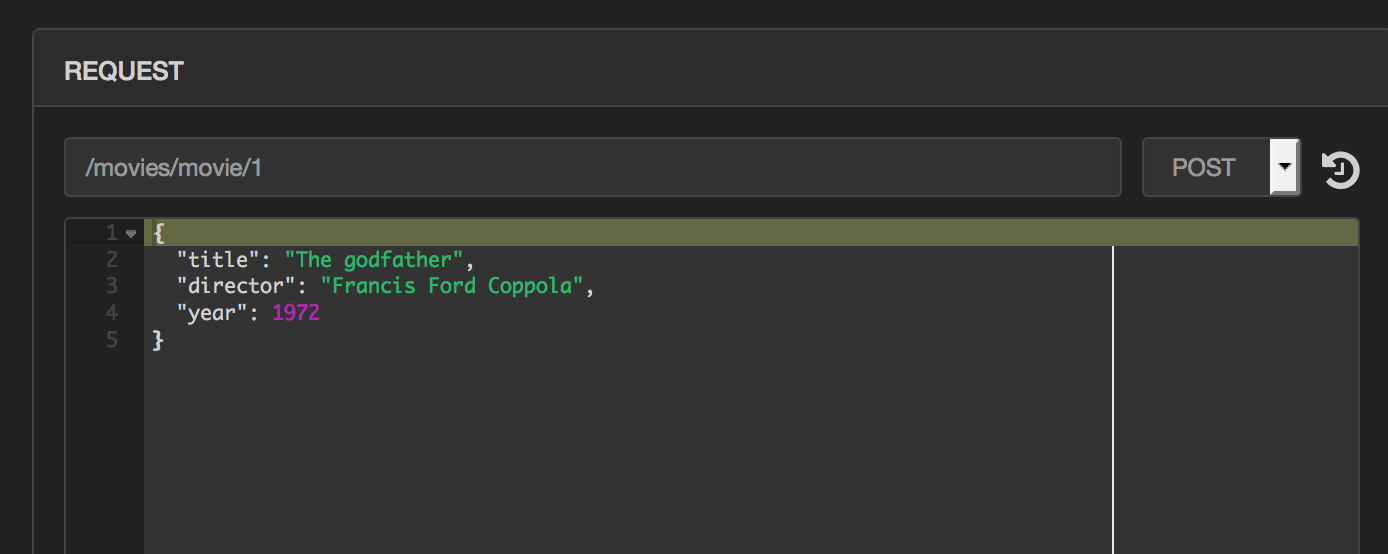
Once you have added a few movies into the index, we can use the same
rest interface in order to search and aggregate data from our index.
Update the path field to /movies/movie/_search. The format of the
path is /INDEX_NAME/TYPE/_search where both INDEX_NAME and TYPE are
optional. If you skip type, your search will apply to all types in the
index, and if you also skip index name, then your search will apply to
all indices.
There are a number of different types of quires that are supported by
ElasticSearch. However, we cover some of the common types here. The
first type of query is the free text query. The query string parameter
allows for fairly complicated queries using the Elasticsearch Query
DS.
However, we can also enter a simple string to match. This will match the
specified word or words in any field in the documents over which the
query applies.
{
"query": {
"query_string": {
"query": "Apocalypse"
}
}
}
For example, the query above will return the result shown below. It
contains details about the time taken to process the query, the shards
that were searched, the total number of results, and then details of
each result.
{
"took": 139,
"timed_out": false,
"_shards": {
"total": 2,
"successful": 2,
"failed": 0
},
"hits": {
"total": 2,
"max_score": 0.5291085,
"hits": [{
"_index": "movies",
"_type": "movie",
"_id": "AVSvEC1fG_1bjVtG66mm",
"_score": 0.5291085,
"_source": {
"title": "Apocalypse Now",
"director": "Francis Ford Coppola",
"year": 1979,
"genres": [
"Drama",
"War"
]
}
}
....
In addition to the query text, you can also specify a field or est of
fields to limit your query to searching a subset of the document. For
example, the search below should return the same result as before, but
will have to look at a subset of the document, and should have faster
performance for larger data sets. There are many other operations.
{
"query": {
"query_string": {
"query": "Apocalypse"
"fields": ["title"]
}
}
}
We can wrap the query string in a filtered object and then specify a
filter to apply on the results of the query. This allows us to retain
the free form search over the initial dataset, but then filters the
results for the specific data we are looking for.
{
"query": {
"filtered": {
"query_string": {
"query": "Apocalypse"
"fields": ["title"]
}
"filter": {
"term": { "year": 1979 }
}
}
}
}
Lastly, another type of query you may run is an aggregation. This is
useful for computing summary statistics about the data. Two examples of
these types of aggregations are shown below. The first will return a
count of the movies directed by each director. The second will return
the average rating for all the movies in our data set.
{
"aggs": {
"group_by_director": {
"terms": {
"field": "director"
}
}
}
}
{
"aggs" : {
"avg_rating" : { "avg" : { "field" : "rating" } }
}
}
Elasticsearch is one of the best ways of running analytics over large
unstructured datasets and is used extensively in many domains from
log-aggregation, machine learning to business intelligence. In this
article, we have looked at how simple it is to set up a fully
functioning Elasticsearch cluster on Rancher using the catalog. In
addition, we have taken a quick look at the power of Elasticsearch using
the rest API. Once you have Elasticsearch up and running you can use it
for a host of different use cases with the many available visualization
and aggregation frameworks such as
Kibana for real time
visualization or Pentaho for business
analytics.